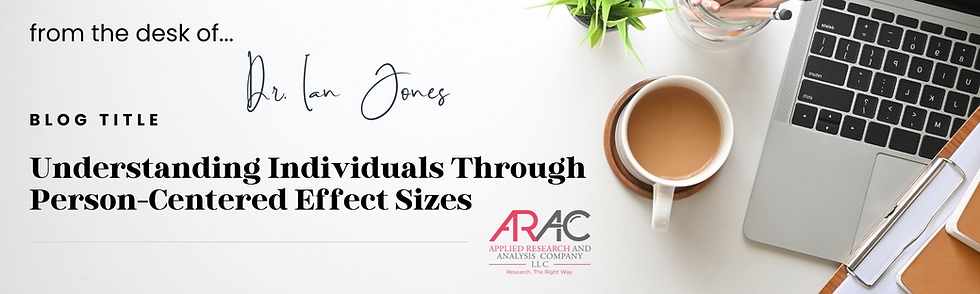
At ARAC, we continue to look for the most relevant design and statistical methods to better answer research questions. While NHST is the industry statistical standard, our scientists have explored alternative avenues to understanding human behavior, specifically through Person-Centered effect sizes. Averages tell us about individual behavior in general, and always with consideration of the group. But to better understand individual behavior and how individuals makeup the greater landscape of a population, it's also important to consider a within-person centered approach to behavior.
Introduction to Effect Sizes
Effect sizes are statistical measures used in research to quantify the importance of a difference found in a study.
Traditionally, these metrics focus on average group differences, associations between variables, or the relative risk, addressing the magnitude of effects rather than their prevalence among individuals.
The Need for a Person-Centered Approach
However, researchers commonly ask: "How many individuals in my study actually behaved or responded in a manner consistent with theoretical expectations?"
Addressing this question requires a shift from traditional effect size indices to a more individual-centric perspective. This shift not only enhances the understanding of data but also democratizes the interpretation of research findings, making them accessible to both scientists and non-scientists.
Person-Centered Effect Sizes: A Practical Innovation
The concept introduced in this paper proposes treating individual responses as effect sizes. This innovative approach involves computing the percentage of study participants whose responses align with theoretical predictions.
For example, alongside traditional metrics like Cohen's d or eta squared (η2), researchers might report that 80% of participants matched the theoretical expectation. This straightforward percentage offers an intuitive understanding of how well a theory performs in real-world settings.
Bridging Theory and Practice
By analyzing several recently published studies, the researchers demonstrate how this method can uncover novel patterns within data.
These insights are important for extending and developing theories, offering a practical tool for researchers aiming to refine theoretical frameworks based on empirical evidence.
Statistical Shifts in Research
The article also references the broader statistical landscape, noting the decline of null-hypothesis significance testing (NHST) in favor of more nuanced inferential methods.
This context highlights the increasing importance of alternative approaches, like the estimation of a priori and statistical-sameness methods, which have gained traction as more robust tools for understanding complex data.
Real-World Application and Case Studies
The paper exemplifies the application of person-centered effect sizes through various studies.
For instance, one study examined the impact of suppressed affective expressions on the perception of neutral faces, revealing that only a minority of participants perceived faces as expected under theoretical conditions. Such findings underscore the utility of person-centered metrics in providing a more accurate picture of individual variability in response to experimental manipulations.
Methodological Considerations and Future Directions
This approach draws on well-established nonparametric statistical methods, making it both easy to compute and generalizable across different types of research designs and variables.
Looking ahead, the researchers suggest that this method could significantly influence future research, encouraging more personalized analyses and potentially leading to more effective interventions tailored to individual responses.
Conclusion: A Call for Broader Adoption
The shift towards person-centered effect sizes represents a critical evolution in the measurement of research impacts.
By focusing on individuals rather than averages, this method offers a more detailed understanding of how research findings apply at a personal level.
As the scientific community continues to embrace these innovative metrics, the potential for more targeted and effective research grows exponentially.
Read the full paper here.
Engaging with the Concept
In the paper, a few specific examples of person-centered effect sizes (PCC, Percent Correct Classification) were detailed. Here are the main points and some metrics:
Siegel et al. (2018) Study:
Study Design: Participants rated facial expressions under conditions of continuous flash suppression. They rated neutral faces shown to the dominant eye, while expressive faces were shown in low contrast to the non-dominant eye.
Outcome: The study found that mean affective ratings aligned with the theory that neutral faces are perceived differently depending on the expression paired to the non-dominant eye.
Person-Centered Metrics: Only 24.44% of participants (11 out of 45) had mean ratings that matched the expected ordinal pattern (positive > neutral > negative). This outcome illustrates the utility of PCC in highlighting how individual responses deviate from or align with theoretical expectations.
Sawaoka and Monin (2018) Study:
Study Design: Participants were exposed to an offensive media post and fictitious comments either in a nonviral (2 comments) or viral (10 comments) context. They evaluated the commenter based on several attributes.
Outcome: The hypothesis was that evaluations would be more negative in the viral condition. Traditional effect sizes supported this prediction, but the interesting insights came from the PCC analysis.
Person-Centered Metrics: Across 38,016 individual comparisons between participants in viral and nonviral groups, only 54.59% had ratings aligning with the hypothesis (viral > nonviral). This shows that while there was a trend consistent with the hypothesis, individual responses varied considerably.
Geniole et al. (2019) Study:
Study Design: This study looked at the effects of testosterone on aggression, analyzing interactions among testosterone levels, personality risk, and genetic markers (CAG repeats) in a competitive setting.
Outcome: Larger effects were seen in individuals with specific combinations of high personality risk and low CAG repeat lengths.
Person-Centered Metrics: When applying PCC to binned categories of behavioral aggression crossed with personality risk and drug condition, only 48.70% of classifications were correct across the whole sample. This percentage increased slightly when focusing on specific subgroups, highlighting the nuanced interplay of factors influencing aggression.
Comentários